What Works, What Doesn’t, What’s Up?
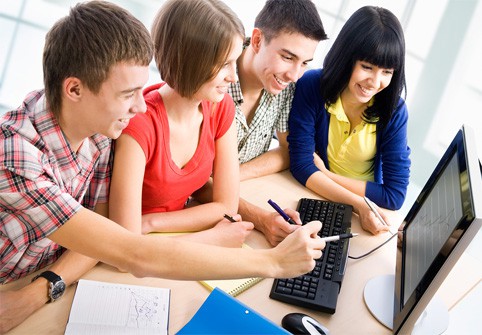
By: Steve Peha
The Common Core and advances in education technology have combined to produce a Big Bang of educational resources. And more teaching and learning tools are coming out every day. The problem now isn’t finding things to work with, it’s finding things that work.
A number of organizations—OpenEd, EdShelf, Graphite, to name just a few—are working not only to provide access to teaching resources but also to sort out what works and what doesn’t. But this is not an easy problem to solve.
Whether people liked NCLB or not, it shifted the dialog on teaching in a profound way by peppering the legislation liberally with the phrase “research-based” over 100 times. From a long-held tradition of “use whatever what you want”, we’ve quickly moved to a place where we value practice supported by scientific research.
But as we discovered through the What Works Clearinghouse effort, good educational research is rare. However, one of those rare moments occurred recently as a landmark study published this year identified clear distinctions between effective and ineffective learning techniques.
The highly useful techniques noted in the study were the following:
-
Practice Testing. Self-testing or taking practice tests over to-be-learned material.
-
Distributed Practice. Implementing a schedule of practice that spreads out study activities over time.
The moderately useful techniques were:
-
Elaborative Interrogation. Generating an explanation for why an explicitly stated fact or concept is true.
-
Self-Explanation. Explaining how new information is related to known information, or explaining steps taken during problem solving.
-
Interleaved Practice. Implementing a schedule of practice that mixes different kinds of problems, or a schedule of study that mixes different kinds of material, within a single study session.
The least useful techniques were:
-
Highlighting/Underlining. Marking potentially important portions of to-be-learned materials while reading.
-
Rereading. Restudying text material again after an initial reading.
-
Summarization. Writing summaries (of various lengths) of to-be-learned texts.
-
Keyword Mnemonic. Using keywords and mental imagery to associate verbal materials.
-
Imagery for Text. Attempting to form mental images of text materials while reading or listening.
Singled out for high use but low usefulness were highlighting, re-reading, and summarizing. This brings us to the real eye-opener in this study: Kids spend more time using less effective techniques than they do more effective techniques. This would be a shocking finding under any circumstance but it’s especially relevant as more and more education technology floods the market.
In his best-selling book, “Why Things Bite Back: Technology and the Revenge of Unintended Consequences”, technology historian Edward Tenner pointed out that “the price of technology is vigilance”. Much like the precious nature of freedom, if we want to continue to get value from technology, and avoid colossal errors, we have to watch how we use it very closely.
Of course, one thing technology can do is gather data on its own effectiveness. But to truly discover what works and what doesn’t, we also have to know what’s up—that is, we need to know the “why” and the “how” of technological advances in education, and we have to measure those advances against yardsticks other than those provided by the creators of the technologies themselves.
What we’re all looking for are replicable results. It’s probably true that just about any technological tool can help a few kids or a few teachers get better results. But if we don’t know why and how those results occur, we can’t easily replicate them at scale—even if scalable technology exists.
This has been a constant challenge in proving out the worth of technology in education since the introduction into schools of the first personal computers. Some things seem to work very well in some situations. But there are so many factors involved in any teaching and learning context that it has been hard to sort out the causal connections between technology use and academic achievement.
More often than not, as specific technologies are researched at larger and larger scales, the statistical effect size of their contributions to learning declines. But if technology developers followed solid research like the study mentioned above, we might make more progress more consistently.
The study in question—really a meta-study based on decades of inquiry into the nature of learning—is one of the best scientific blueprints for research-based practice that we’ve ever had. And many of its findings are ideally suited to technological implementations.
Two of the most effective practices identified in the study—distributed practice and interleaved practice—are often challenging for human beings to pursue by analog means because they require a level of awareness that is hard to maintain while most of our brainpower is being spent actually doing work. But this instructional overhead can easily be offloaded to technology.
Another important finding was the value of low- and no-stakes practice testing. Despite the national backlash against testing, it turns out that testing is good for learning—as long as the testing context isn’t dominated by the pressures that typically accompany the high-stakes state testing that so many people seem so troubled by.
Technology is uniquely suited to this kind of practice. Adaptive learning software that can tailor the work kids do to their individual needs gives them both practice and testing with the crucial assessment information being gathered and applied in the background. It is the hope of many education technology developers that high-interest content combined with adaptive delivery will be the silver bullet that makes learning both fun and effective.
The one big thing we don’t know much about, and which the study does not address, is the social component of learning. How much of what kids learn has to do with their interactions with teachers and other students in the same physical location? Many people believe this is significant but this effect has yet to be quantified conclusively. At the same time, technologies, particularly multi-participant video technologies, get better all the time. Again, however, whether video classrooms are as effective as traditional classrooms is something we have yet to determine.
The challenge of knowing what works, what doesn’t, and what’s up is something that educators and scientists have struggled with for generations. Education technology seems like a natural way to investigate this issue. But solid clinical research, of the quality found in the study referred to here, will likely play a role as well. Machine learning looks more promising all the time but the human element in knowledge and skill acquisition will always be an important factor in success, especially when we think about replicating results at scale.
Steve Peha is a learning strategist and education technologist with more than 25 years of experience. He is the founder of Teaching That Makes Sense, an education consultancy focused on literacy, leadership, and school-wide change. He has written extensively about teaching, technology, and education policy for sites like The Washington Post, The National Journal, Edutopia, and others. He is also the originator of The Agile Schools Project, which he started three years ago with an article that outlined how the popular Agile software methodology could be translated to education. He speaks regularly about this at venues such as Google and Yahoo, and at Agile conferences throughout the US.
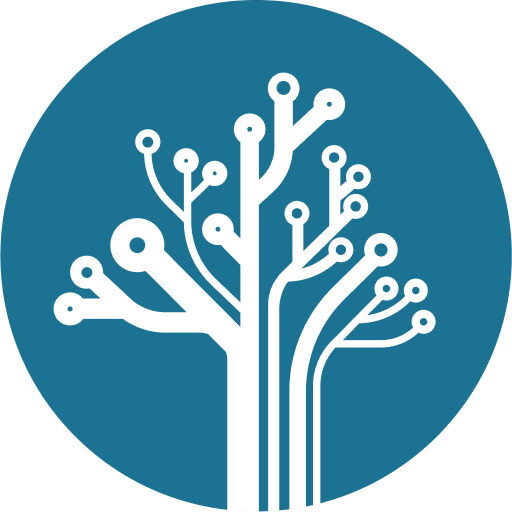
0 Comments
Leave a Comment
Your email address will not be published. All fields are required.