Dr. Tim Dasey on Wisdom Factories and Turning the Educational Model Upside Down
Key Points
-
Education systems should emphasize experiential learning and adaptability over rote memorization to prepare students for a rapidly evolving AI-driven world.
-
Schools need to adopt agile methodologies, allowing for quick experimentation and adaptation to effectively integrate AI and other technologies into teaching practices.
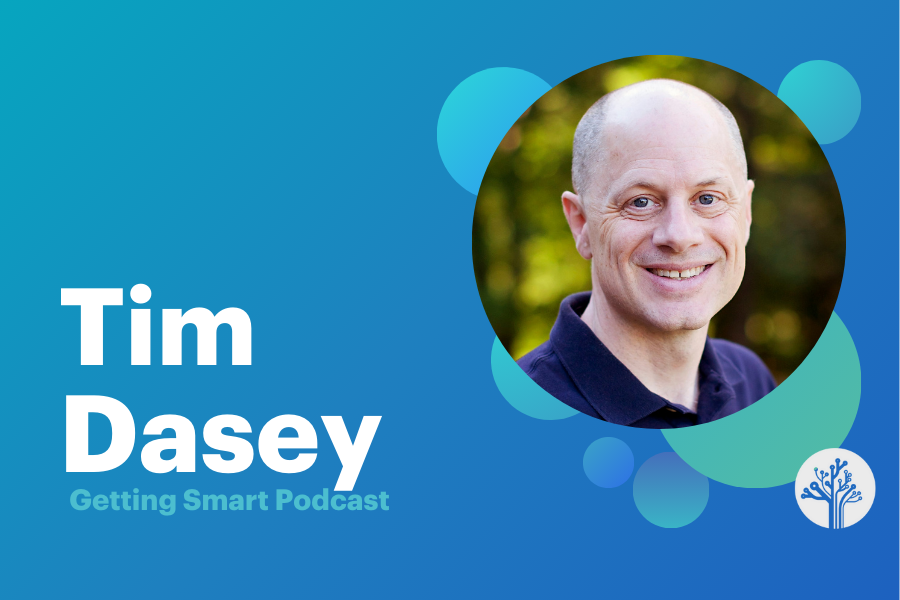
Join Digital Promise and Verizon Innovative Learning on Thursday, November 14, for the third annual Elevating Innovation Virtual Conference. Register for free at digitalpromise.org/elevatinginnovation.
In this episode of the Getting Smart Podcast, Nate McClennen and Tim Dasey explore the multifaceted impact of AI on education, exploring both the challenges and opportunities it presents. As AI becomes a larger part of various educational contexts, it prompts a reevaluation of traditional teaching methods and calls for the creation of new strategies to ensure students are prepared for an AI-driven world. Tim Dasey, drawing on his extensive experience in AI and education, emphasizes the need to shift from rote memorization to experiential learning approaches that foster critical thinking, adaptability, and problem-solving skills. This transformation is crucial for what Dasey refers to as “wisdom factories,” where students not only acquire knowledge but also develop the intuition and judgment necessary to apply it effectively in diverse situations.
The discussion highlights three innovative strategies for enhancing educational practices: integrating games to build experience and intuition, adopting “upside-down learning” that prioritizes problem-solving over traditional subject compartmentalization, and implementing “productivity therapy” to tailor AI tools to individual learning and teaching styles. By embracing these approaches, educators can create dynamic learning environments that encourage experimentation and adaptability, essential traits in a rapidly changing technological landscape. The conversation also touches on the importance of agile methodologies in education, advocating for iterative cycles of implementation and feedback to keep pace with evolving educational technologies and methodologies. Together, these insights provide a roadmap for educators and school leaders seeking to navigate the complexities of integrating AI into educational settings effectively.
Nate McClennen: Hello, everybody. You’re listening to the Getting Smart Podcast, and I’m Nate McClennen. I’m super excited for today’s conversation.
Nate McClennen: Today, we’ll dive into our favorite topic, AI and education. We’re tackling a fundamental question: how is AI going to impact teaching and learning, or conversely, how might teaching and learning impact AI? And, of course, we’ll explore questions that bridge both perspectives.
When you look at the media and talk to people in or outside education, there’s a continuum of perspectives—from doom and gloom, where people worry AI will end the world, to extreme optimism, where others think AI will save the world. There are countless opinions in between, and today, we’re aiming to explore where education fits into this spectrum. Education itself is varied in implementation—some are dealing with policy, others with concerns over AI being used for cheating, or as a tool for efficiency. Then there are forward-thinking folks asking, “What do we do next with AI, and how can it truly transform education?”
AI’s usage is increasing, with nearly every student having heard of AI in some form, and we believe over 50% are using it in some way, even if their school hasn’t officially adopted it. In parallel, billions of dollars are being invested into AI products outside of education. Yet, there’s far less investment in exploring how education itself can be rethought to suit an AI-driven world—how we teach, assess, design curriculum, and prepare young people to thrive alongside AI.
Nate McClennen: Fortunately, today we’re joined by Tim Dasey, who has spent 35 years in AI. It’s a reminder that AI existed before ChatGPT! Tim spent 30 years at MIT’s Lincoln Laboratory, working on national security programs, technology, and AI, among other fields. Last year, he wrote a fascinating book titled Wisdom Factories: AI, Games, and the Education of a Modern Worker. Tim, welcome—we’re thrilled to have you with us.
Tim Dasey: Thank you. I’m pleased to join you.
Nate McClennen: We’re going to have a wide-ranging conversation today, which I think will be interesting since both of us love this topic. I’d like to start with an easy question, which has two parts. First, what did you want to be when you were in high school? And second, what was the most inspiring learning experience you had between birth and college graduation? These questions will help our audience get to know you a bit better.
Tim Dasey: I grew up during the space race. In the early 1970s, teachers would wheel TVs into the classroom so we could watch the launches and see the steps taken on the moon. So, physics was always in my head, but honestly, by the end of high school, I still had no clear idea of what career choices were available. One of my friends in senior year mentioned he was going to study engineering, and I didn’t even really know what that meant. Life can take some unexpected turns, though.
Regarding your second question, one of the reasons I took the path I did—which led to electrical engineering, then computer engineering, biomedical engineering, and finally work that crossed over into multiple disciplines—was an experience I had in my senior year of high school. In 1981, I took a one-semester computer programming class using a RadioShack TRS-80 with 16 kilobytes of memory and a cassette deck for storage. There were maybe half a dozen students in the class, it was entirely self-paced, and project-oriented. It really captured my imagination. That one teacher was very forward-thinking—when you consider that, even today, half of U.S. high school students graduate without taking any computer courses.
Nate McClennen: I remember those days! I have this image in my mind of a dark screen with green or white text, using basic code with lines like 10, 20, 30, and so on. I have two children in college now, and they grew up in a completely different world, where they’re fully immersed in technology and the internet. We didn’t have email back then, and we were only just beginning to think about coding. Now, they’re facing an entirely new challenge with the arrival of AI. Given your long experience in AI, how has the concept of AI evolved since you first started studying it 35 years ago? For our audience of educators, school leaders, and district leaders, what should they know about the mechanics of AI?
Tim Dasey: I became involved with the first generation of artificial neural networks, which remain the foundation for today’s most powerful AI systems. I started working with neural networks in the late ‘80s and early ‘90s, but my perspective was very different from today’s. I was working in a lab that studied mammalian visual systems, using neural networks to model biological systems and later to process neurological signals. Interestingly, a lot of the math that underpins AI today already existed back then. We’ve seen tremendous scale-ups and innovation since, but the core concepts have stayed the same.
A key misunderstanding among educators is that they often focus on incorporating AI into the educational process. But in my recent work, especially since publishing my book, my focus has been on teaching about AI. When you step back and consider what’s involved in getting a machine to “learn” and “think” (using those terms loosely), you realize that many of the underlying principles mirror human learning and thinking processes. Teaching about AI involves teaching about learning, thinking, and the work and cognitive processes humans go through. I believe the real value lies in comparing these processes throughout the educational journey.
Nate McClennen: That’s a fascinating perspective, and one we’ve been exploring too. I imagine an AI learning process diagram next to a human learning process. Both systems take in inputs—we receive sensory inputs, while a large language model receives text-based inputs. Both process these inputs—our brains process them into thoughts, while an AI model processes them through algorithms. Then, both produce outputs. In your view, what’s the fundamental difference between the two, even if the processes appear similar?
Tim Dasey: I think the main difference is scale. Back then, I was working with networks of small mathematical units that we called “neurons” because they share some similarities with biological neurons. My networks might have had a few hundred neurons. By comparison, GPT-4, for example, reportedly has around 1.8 trillion connections among billions of neurons. So, what I was working with was essentially the equivalent of a cockroach brain, while today’s systems are comparable to whales or apes in terms of complexity and capabilities.
Another significant change relates to the scope of the tasks. Whereas earlier AI models were designed for narrow applications—like reading medical images—modern AI models like ChatGPT were trained on much more generalized tasks. ChatGPT’s training involved predicting and generating the next “character” or token in response to an input. For it to perform this task effectively, the model had to learn an array of complex concepts to transform those inputs into meaningful outputs. This generality wouldn’t have been possible in earlier models because the networks were too small to handle such complexity.
Building Wisdom Factories: Skills for the Future
Nate McClennen: Let’s talk about your book, Wisdom Factories. I love the opening quote from Isaac Asimov: “The saddest aspect of life right now is that science gathers knowledge faster than society gathers wisdom.” Why did you choose this quote in the context of AI acceleration?
Tim Dasey: That quote resonates deeply with the personal transformation I’ve experienced in my career. It reflects my shift towards education and teaching. Despite working at a university for over 30 years, I was on the technology and research side, not on the academic side. Moving into education required a leap of faith, as much of my past work, done for national security, was confidential and couldn’t easily be shared. In a way, Asimov’s quote describes my realization that AI would keep advancing with or without me. The problem is this imbalance of power. I’ve been speaking more about this recently in the context of education. If I’m smarter than an AI tool, I can evaluate its output, use it effectively, and leverage it as a complementary tool. But if the AI is much smarter than me, then I’m essentially at its mercy, much like I would be when dealing with an expert human in a field I don’t understand. This dynamic raises an essential paradox in AI education: we want to help students reach a point where they can critically analyze the information they encounter, but the learning process itself may require that they aren’t just relying on AI to provide the answers.
When I saw AI beginning to automate even the work of AI engineers, I became concerned about this imbalance. The question became: can humans step up to take on more challenging cognitive roles in an age where AI can perform high-level tasks that were once beyond reach for most people? That led me to explore whether our educational systems are prepared to teach the skills needed for that level of adaptation.
Nate McClennen: Before we get into how to build these skills, let’s talk about what skills we need. As AI accelerates and takes on more tasks, what unique or critically important skills do humans need to focus on? What does it mean to create a “wisdom factory,” as opposed to an “expertise factory”?
Tim Dasey: The term “wisdom” might not be perfect, but it represents a different approach to education. Instead of building knowledge brick by brick, where students accumulate details that lead to abstract concepts, I advocate for an experience-based approach that fosters intuition. In this model, skills aren’t just acquired through knowledge transfer; they’re built through practice, experience, and reflection. The goal is to develop skills that are more versatile and adaptive, grounded in intuition rather than rote learning.
The traditional system focuses on building specialized expertise—where students narrow their focus until they become experts in a particular field. And we’ll still need experts; for example, nurses must know how to perform their job. But their work could look entirely different in five or ten years. In fast-evolving fields like AI, the knowledge learned today might be obsolete within a year or two. So instead of filling people’s heads with static information, we should help them develop skills that allow them to adapt to new information and challenges as they arise.
This approach emphasizes interdisciplinary thinking, experiential learning, and the development of judgment and intuition. Rather than focusing solely on acquiring knowledge, the goal is to prepare students to navigate complex, evolving problems.
Nate McClennen: I like that distinction between building specialized expertise and cultivating a “wisdom factory.” But I have to push back a bit: is there some core knowledge that everyone should have, a foundation that remains essential?
Tim Dasey: Absolutely. I’m not saying that people don’t need detailed knowledge. But rather than learning information for “some day,” I suggest gaining knowledge as needed to solve real-world problems. We know that the brain retains information best when it’s used regularly—”use it or lose it” is a principle that applies down to individual neurons.
Take STEM education, for example. Students spend years learning science, technology, engineering, and math, but they’re rarely given a complex, open-ended problem that requires them to decide which concepts and techniques apply. In high school, my trigonometry teacher gave us extra credit if we participated in a math competition, and the questions in that competition were unlike anything I’d seen before. They didn’t even look like typical math problems; the main challenge was figuring out what type of math to use. That kind of decision-making skill is much more relevant in today’s workforce, where people encounter novel problems regularly.
So yes, there is a core, but it’s not static. It’s more about developing the ability to identify and access relevant information quickly, rather than memorizing it all upfront. The real skill lies in knowing how to navigate information, understand context, and make decisions based on that.
Nate McClennen: It’s not algorithmic anymore. Let’s pivot to what the new system looks like. In your book, you propose three main strategies: games, turning the educational model upside down, and something called productivity therapy. Could you walk us through these?
Tim Dasey: Sure. Let’s start with games. This isn’t a magic bullet, nor is it solely about computer-based learning; games can be as simple as playground activities. The idea is to give students a challenge, let them attempt it, fail, learn from their mistakes, and try again. It’s about iterative experience and safe spaces to fail without the real-world consequences. Life doesn’t always afford us enough opportunities to build well-rounded experiences, so games serve as a controlled environment where students can develop skills through practice.
Nate McClennen: We spend a lot of time in education trying to provide students with real-world experiences. But you’re suggesting that games could serve as a supplement. Is that correct?
Tim Dasey: Exactly. Even adults benefit from this. The difference between someone who can handle a wide variety of situations and someone who can’t often comes down to experience. Those who have faced unusual situations tend to be more adaptable. Games allow us to simulate these situations and expose people to various scenarios without real-life consequences.
For instance, we used to conduct games with professionals from the military, homeland security, public health, and other sectors. We would play out scenarios that evolved over time, accelerating events that might unfold over weeks in real life so that participants had to make quick decisions. We focused on key judgments they would need to make, then introduced variations on those judgments to build adaptability. By compressing time and adding variations, we could cover 15 to 20 cases in an hour. Games fill a niche that can’t be fully addressed by field exercises or textbook learning. Historically, creating games was challenging and time-consuming, but technology has made it much easier now. The second strategy I propose is what I call “upside-down learning.” Essentially, many of our instincts about how to educate students are backward. When trying to build intuition, the best approach is to start with experiences and then have discussions that build on those experiences, rather than frontloading students with information and hoping it sticks.
One problem is that traditional schooling isn’t structured to support this. In most schools, subjects are compartmentalized—chemistry is taught separately from physics, which is taught separately from biology, and so on. But in real life, problems don’t fit neatly into one discipline. Imagine giving students a problem like antibiotic-resistant bacteria. To address it, they’d need to learn concepts from biology, chemistry, and maybe even sociology or psychology. By giving students problems that span multiple subjects, they can engage in meaningful learning dives as they explore the knowledge needed to tackle the problem.
Another benefit of this approach is that it builds transferability by helping students see connections between fields. For instance, the skills required to analyze a disease outbreak may be similar to those needed to analyze a security threat or even social media trends. When we structure learning around real-world problems, students develop the ability to transfer skills and knowledge across contexts. But this approach doesn’t fit neatly into a traditional school schedule, where classes are divided by subjects. Interdisciplinary classes exist here and there, but they’re not common enough to support widespread problem-based learning.
Nate McClennen: I completely agree. We see schools experimenting with project-based or problem-based learning, but it’s difficult to mainstream those methods within the existing framework of subjects and Carnegie units, especially in public secondary schools that have strict credit requirements. Turning the system upside down would require a complete restructuring.
Let’s talk about your third strategy, which you call “productivity therapy.” Can you explain what that means?
Tim Dasey: Productivity therapy is essentially what teachers are going through right now as they figure out how to integrate AI into their classrooms. Generative AI, like large language models, is a long-tail technology, meaning its value lies in meeting a wide range of specific needs. But those needs vary significantly, even from one teacher to the next. Some teachers need help with organization, while others need tools to capture their ideas quickly or streamline grading. Each person has a unique approach to their work, and AI can be customized to fit individual needs.
What I mean by “productivity therapy” is the process of understanding one’s own cognitive and working style to choose the right tools. Think of it like going to therapy to understand yourself better, but in this case, it’s about understanding how you work and where AI can enhance your productivity. As an ADHD person, for example, I use tools that help me stay organized, capture ideas when I’m on the go, and stay focused.
The issue is that each teacher or professional needs different types of support, so a one-size-fits-all solution doesn’t work. What we need are support systems that allow teachers (or any professional) to experiment with different tools and see what works for them. This process can be iterative, with regular feedback and adaptation, similar to therapy in a way, but focused on productivity.
Nate McClennen: So, productivity therapy is really about helping individuals customize their workflow, particularly in light of AI’s capabilities. It’s an interesting idea. Let’s shift focus a bit and talk about broader education recommendations. The landscape right now is chaotic. Some schools are focused on policy, some on implementation, some on preventing cheating, and some on making things more efficient. It’s understandable, given how fast AI has arrived on the scene. What would you recommend as the most important first steps for school leaders and educators?
Tim Dasey: I’d recommend three basic principles. First, people need experience. If you want to understand what works, you need to try different things, and that means allowing some tolerance for error. Not every experiment will succeed, but it’s important to create a culture where experimentation is encouraged.
Second, there should be mechanisms for sharing lessons learned. Schools need platforms and communication channels that allow educators to discuss what’s working and what isn’t. This sharing of insights is crucial, especially in such a fast-evolving field.
Third, schools need to adopt a more agile approach. In the software industry, we use a process called Agile development, where we build a little, test it, and adjust based on feedback. This iterative approach is essential in a world where things change quickly. Schools often have multi-year timelines for implementing new programs, but by the time they’re ready, the world has moved on. Instead, we need to focus on quick cycles of implementation and feedback.
This approach requires a cultural shift, as schools are traditionally cautious about taking risks with students. But students aren’t widgets—they’re human beings who need to be prepared for a world that’s constantly changing. Allowing teachers and students to experiment and make adjustments along the way is essential if we’re going to prepare them effectively for the future.
Nate McClennen: Absolutely. The schools that embrace innovation tend to give teachers more autonomy and encourage networking to amplify learning across their communities. Education has struggled with agile processes; we tend to plan large programs and let them run for years before making changes, which doesn’t align with today’s pace of change.
All right, Tim, last question. What are you working on next? You published a book last year, left MIT, and now you’re consulting. What’s on the horizon for you?
Tim Dasey: I’m currently balancing consulting, speaking engagements, and professional development strategy for K-12, corporate, and higher ed audiences. But I have two major projects in the works. The first is a new book, which will be a practical guide for educators on AI. It will cover everything from managing cheating to teaching about AI, as well as pedagogical and structural considerations for integrating AI into classrooms.
The second project is developing a comprehensive AI curriculum for K-12. I’m designing it to be highly flexible and modular, so schools can adapt it to fit their specific needs. One of the exciting things I’m exploring is using AI to help customize the curriculum, creating different pathways depending on the school’s capacity and the needs of its students.
Nate McClennen: That’s exciting! We really need that, especially a curriculum that emphasizes wisdom and adaptability over pure information transfer. Thank you, Tim, for sharing your insights with us. Let me quickly summarize what we discussed today.
We started with the concept of a power imbalance in AI and the question of whether humans can rise to the challenge of a world increasingly shaped by AI. From there, we explored the idea of “wisdom factories” versus “expertise factories” and discussed three strategies for building these skills: games, upside-down learning, and productivity therapy. You also highlighted the importance of tolerance for experimentation and the need for agile processes in education. Finally, we discussed your current work, including a new book and a K-12 AI curriculum.
For our listeners, you can find Tim online at timdasey.com or connect on LinkedIn. He also writes a Substack newsletter called Sweet Grapes—that’s “GRAIPES” for the AI pun—where he publishes weekly articles. We’ll put these links in the show notes for easy access. Thanks again, Tim.
Tim Dasey: Thanks, Nate. It’s been a pleasure.
Nate McClennen: Be sure to check out Tim’s book on Amazon and subscribe to his newsletter. Thanks to everyone for listening to this episode of the Getting Smart Podcast.
Dr. Tim Dasey
After receiving his PhD in biomedical engineering, Tim Dasey launched a 30-year career with the MIT Lincoln Laboratory, a government-funded laboratory that researches and develops technology that supports national security. Today, he is an author and strategic educational consultant, whose first book, Wisdom Factories: AI, Games, and the Education of a Modern Worker, came out in June 2023. It draws on his experience with AI, to propose a new educational model that trains modern workplace leaders by focusing on wisdom skills such as critical thinking and relationships as AI increasingly becomes a source of workforce expertise.
Links
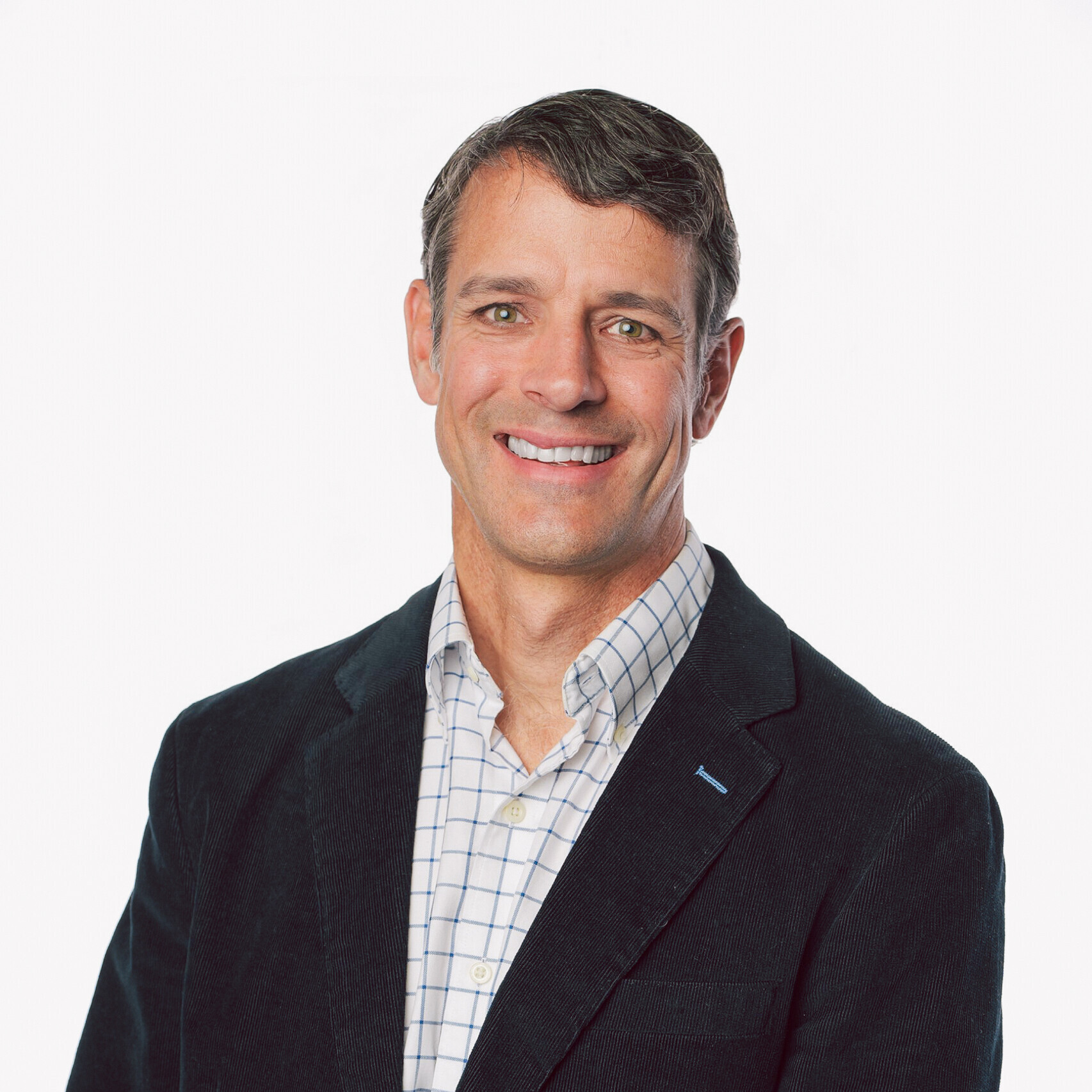
0 Comments
Leave a Comment
Your email address will not be published. All fields are required.