Probably Approximately Correct
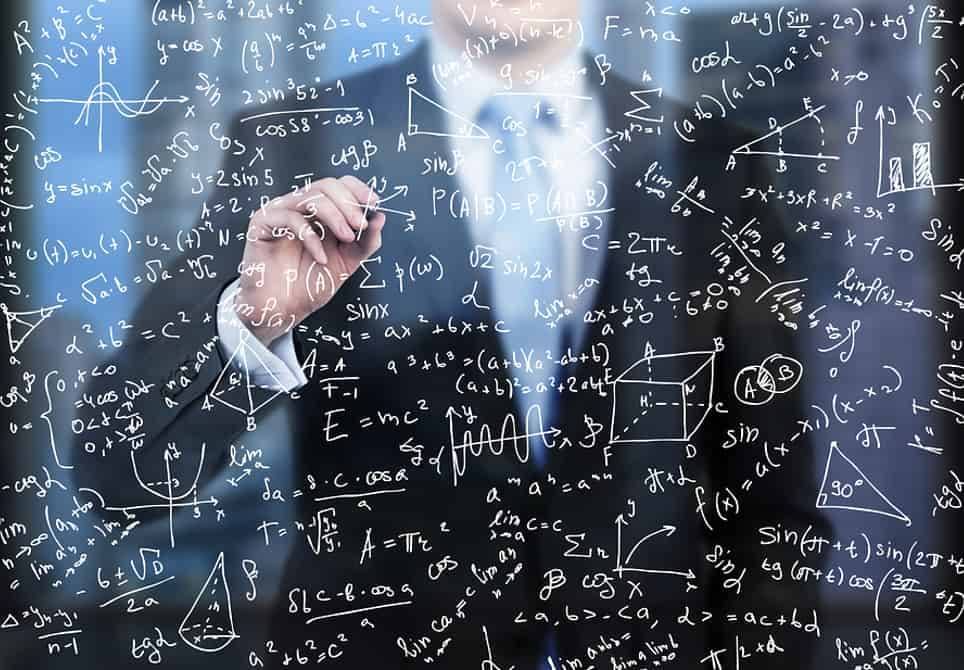
The best we can hope for when it comes to most decisions is to be probably approximately correct–a high probability of being about right.
In finance, analysts compare proposed capital costs with discounted anticipated future cash flows to calculate a net present value–a bunch of assumptions with the hope of being probably approximately correct.
Insurance is a hedge against a big loss; it’s based on the probability of bad stuff happening–the insurance company makes a little money if their calculations are probably approximately correct. A doctor takes a few data points and makes a diagnosis hoping she is probably approximately correct. School facilities planners estimate future enrollment trends and then school boards estimate the likelihood of a community support for a construction bond, both hope to be probably approximately correct.
It wasn’t until the recently that these human analysts could be aided by smart machines that could learn from big data sets and improve predictive power. But it turns out that nature has learning all along–natural selection wasn’t just dumb luck, it was bio-algorithms that learned by interacting with the environment.
A Valiant Attack on Complexity
In machine learning, probably approximately correct learning (PAC learning) was proposed by Harvard professor Leslie Valiant 30 years ago as way of dealing with computational complexity. Learners (people or algorithms) develop a hypothesis with the high probability (the “probably” part) of having a low generalization error (the “approximately correct” part)–a measure of how well an algorithm can predict outcomes for a new data set.
In Valiant’s 2013 book, Probably Approximately Correct: Nature’s Algorithms for Learning and Prospering in a Complex World, he offers a grand unifying theory of how life evolves and learns.
Valiant’s study of evolutionary biology led him to conclude that natural selection was directionally correct but couldn’t explain the rate at which evolution occurs: “At present the theory of evolution can offer no account of the rate at which evolution progresses to develop complex mechanisms or to maintain them in changing environments.”
More than random selection, Valiant argues, evolution is driven by “ecorithms” which incorporate information gathered from the environment to improve an organism’s performance.
Valiants book is based on two central tenets, “The first is that the coping mechanisms with which life abounds are all the result of learning from the environment. The second is that this learning is done by concrete mechanisms that can be understood by the methods of computer science.”
For more computational learning theory, listen to Valiant’s Harvard colleague Ryan Adams discuss PAC learning and check out 9 Ways Smart Machines Are Improving Your Life.
Stay in-the-know with all things EdTech and innovations in learning by signing up to receive the weekly Smart Update.
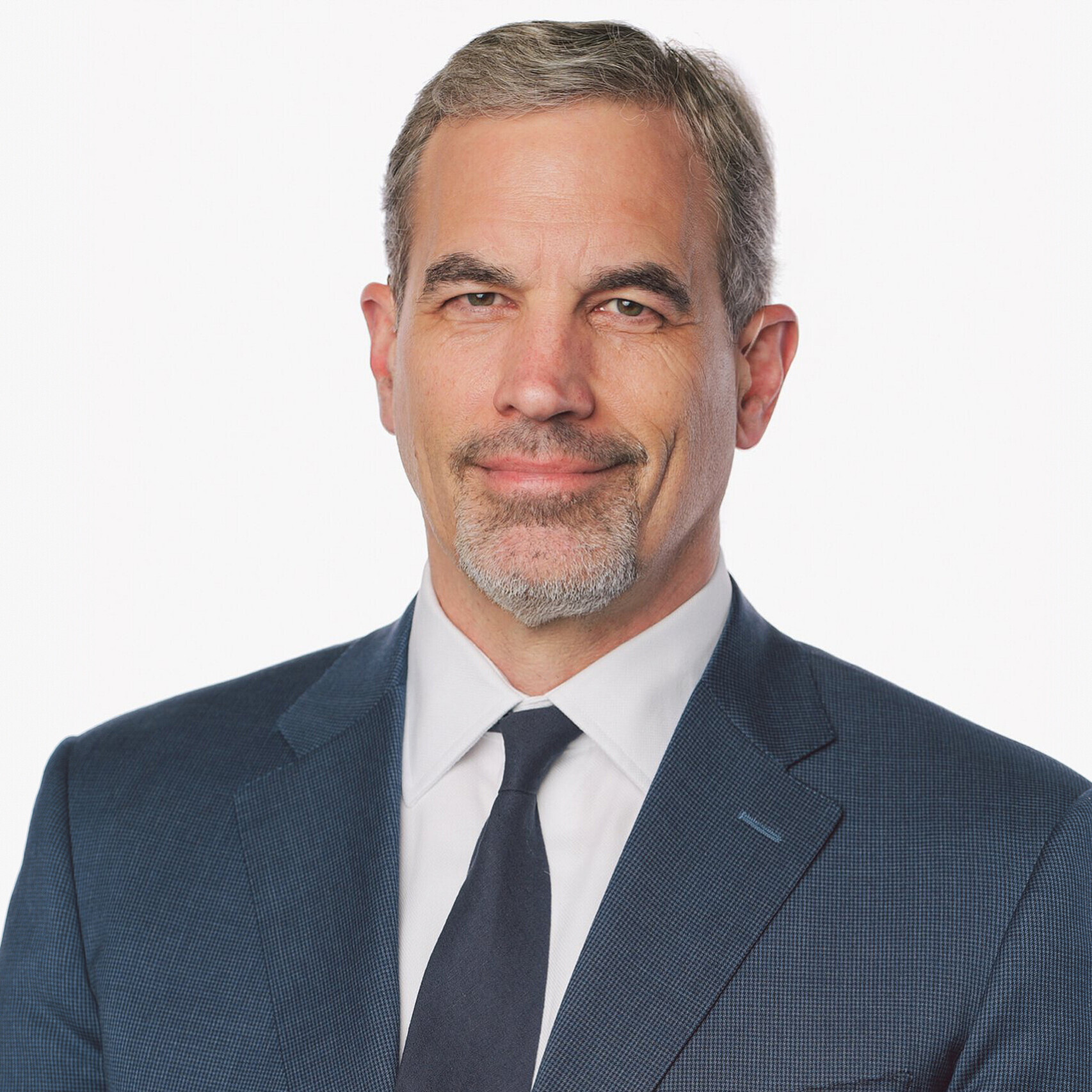
0 Comments
Leave a Comment
Your email address will not be published. All fields are required.